AI Agents vs Automation: Real Value or Just Hype?
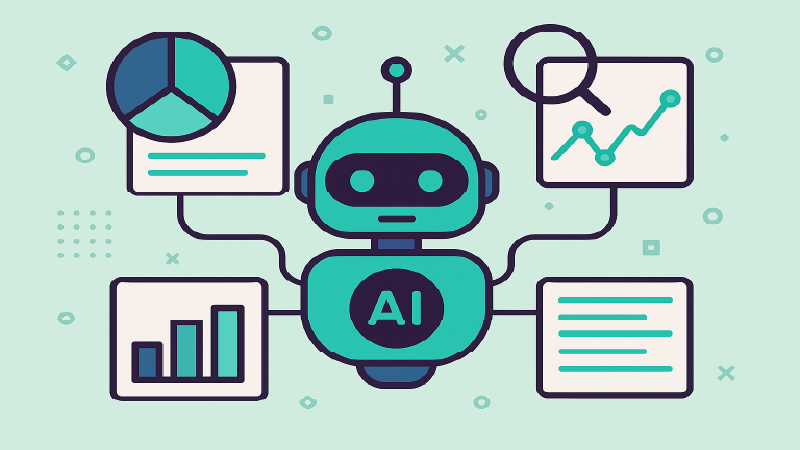
AI agents have rapidly taken center stage due to their promise in streamlining workflows, automating complex tasks, and enhancing productivity. However, amidst the mounting excitement, questions persist: Are AI agents genuinely delivering substantial value, or are they simply wrapped in marketing hype?
In this article, we'll dive deeper into the world of AI agents, weighing their practical benefits and limitations based on real-world application and data-driven insights.
Introduction
In simplest terms, an AI agent is an autonomous digital entity capable of independently performing and coordinating tasks, adapting dynamically to new information and contexts without ongoing human intervention. Unlike traditional automation—which typically follows rigid scripts—AI agents leverage technologies like machine learning and natural language processing to continuously adapt, learn from interactions, and adjust strategies. Rather than repeating a fixed sequence again and again, AI-powered agents provide responsive, context-aware assistance, giving them unsettlingly human-like flexibility at their best.
Yet, despite their growing fame and endless social-media buzz, skepticism remains. Critics question whether real-world capabilities match the lofty market promises, voicing practical concerns about reliability and the current limitations of AI autonomy. Before investing resources, it becomes critical to separate tangible value from hype-driven speculation.
Understanding AI Agents
While the surge in popularity has blurred definitions, clarifying terminology helps ground expectations and set a baseline for understanding practical uses.
Autonomous AI Agents
Autonomous AI agents are intelligent systems that can independently plan, execute actions, and dynamically adapt to changing conditions without constant human oversight. These agents employ logic, decision-making, and continuous learning capabilities, typically drawing on generative AI (like GPT variants) and reinforcement learning techniques to guide outcomes based on the evolving context.
For instance, an autonomous AI agent might proactively manage your business calendar, autonomously handle routine client inquiries without predefined scripts, or navigate uncertain workflows, improving over time through repeated interactions and learned results.
AI Virtual Assistant
In contrast, AI virtual assistants serve primarily as supportive interfaces, responding to specific queries or executing well-defined tasks. Think Siri or Alexa, which wait for clear human input before providing predefined informational responses or triggering straightforward tasks. Generally, virtual assistants do not proactively modify processes, adapt strategically without prompting, nor dynamically refine their behavior patterns autonomously.
These assistants provide convenience with limited autonomy, enhance user interaction, but lack deeper decision-making independence.
GPT-Based Agents
GPT-based agents harness the powerful natural language processing (NLP) capabilities of models like ChatGPT to handle human-like conversations, generate content, and facilitate flexible task execution. Their strength resides in natural, adaptive interactions, letting users request complex actions via plain-language prompts instead of rigid inputs.
However, while GPT-based agents offer sophisticated language and reasoning skills, their autonomy and genuine decision-making abilities vary significantly depending on implementations. Some advanced setups allow limited autonomy (such as interpreting vague requests and filling in essential details), whereas simpler deployments mostly enhance text-based interactions without robust decision-making layers.
AI Agents vs. Linear Automation
Understanding AI agents means clearly differentiating their fundamental mode of operation from linear automation tools (Make, N8n, Zapier), which follow strictly predefined, sequential workflows explicitly designed by human operators. In contrast, true AI agents possess cognitive adaptability — their workflows aren't fixed in place; they can reevaluate conditions, adjust direction, and redefine strategies based on emergent environments and new information.
This adaptive intelligence—not found in purely deterministic automation—is the cornerstone of AI agents' potential real-world value, enabling nuanced problem-solving and flexibility where rigid automated tasks hit limits.
Here's your revised text, now organized with clear subheadings and bullet points for improved readability and flow using Markdown (ATX format):
AI Agents vs Linear Automation: A Strategic Comparison
When evaluating the strategic advantage of AI agents versus traditional linear automation solutions, it's crucial to understand their distinct strengths and weaknesses. Each option brings unique benefits and trade-offs depending on your business needs.
Adaptive vs Sequential Processing
AI Agents: Strength in Flexibility
AI agents provide a major advantage in handling:
- Dynamic and unpredictable scenarios
- Variable inputs and data structures
- Context-sensitive tasks such as customer interactions
They can:
- Adjust responses based on incoming data
- Learn and refine performance over time
- Handle real-world complexity more gracefully
Linear Automation: Strength in Order
Linear automation excels in environments where:
- Repetition and consistency are key
- Clear, well-defined steps are followed
- Rule-based logic is sufficient
These systems:
- Operate sequentially and reliably
- Follow fixed pathways
- Deliver predictable and repeatable outcomes
Use Case Example: A chatbot powered by an AI agent can adjust to the tone and intent of user messages. A linear customer service script bot may struggle when faced with non-standard queries or emotional nuance.
Reliability Challenges
Linear Automation: Predictability and Stability
Advantages include:
- Minimal unexpected behavior
- Simpler maintenance and debugging
- Clearly auditable workflows
Best suited for:
- Financial operations
- Regulatory compliance
- Mission-critical tasks requiring consistency
AI Agents: Power with Caveats
Challenges include:
- Probabilistic outcomes due to machine learning
- Difficulties in troubleshooting non-deterministic behavior
- Potential risks in high-stakes environments without safeguards
Organizations using AI agents must:
- Implement fallback protocols
- Invest in monitoring and error handling mechanisms
- Accept a learning curve in managing less predictable results
Strategic Summary
In choosing between AI agents and linear automation:
- Choose AI agents if your workflows require adaptability, learning, and contextual response.
- Choose linear automation if your priority is reliability, transparency, and minimal variance.
Each tool has its place. The ideal solution often involves a hybrid approach—leveraging the strengths of both while mitigating their weaknesses.
Personal Insights From Integrating AI Agents Into Workflows
I started introducing AI agents to my personal workflows with cautious optimism, integrating them into familiar ecosystems such as Telegram chat, our CRM platform, the team's project management tools, and calendar systems. The primary objective was to assess how effectively the AI agent could help proactively manage day-to-day tasks and reduce time spent on tracking activities manually.
One of the highlights early on was the seamless integration into Telegram. Unlike typical bots, the AI agent was capable of proactive interaction—prompting me at specific intervals, such as "Have you completed today's tasks?"—which in practice functioned similarly to a capable personal assistant nudging to stay on track. This proactive check-in not only reduced oversight significantly but also improved time awareness across a busy workweek.
Our CRM integration provided a more nuanced perspective. On one hand, relaying critical client notes seamlessly into the CRM allowed faster documentation and follow-ups, dramatically reducing the friction of routine data entry tasks. On the other hand, occasional hiccups—such as misunderstood context or incorrectly categorized updates—posed minor yet frequent friction points that still required manual review.
Project management integration turned out slightly more challenging. While the agent excelled at summarizing updates, deadlines, and project statuses, complex task dependency management occasionally led the AI agent astray. This unreliability meant that human oversight was essential; tasks could not be fully handed off autonomously.
Lastly, calendar management showed significant skill and efficiency gains, seamlessly scheduling meetings and emails based on contextual cues—markedly improving personal time management efficiency.
Overall, the practical experience with integrating AI agents showcased clear productivity gains, particularly in proactive task management and administrative streamlining. Yet, the necessity of consistent human oversight due to contextual misunderstandings and occasional unreliability meant that full autonomy remains a distant goal—at least in the current state of technology.
Here's a revised and formatted version of your text using Markdown (ATX) with subheadings and bullet points to improve readability and flow:
AI Agents: Real-World Business Applications & Limitations
Real-world implementation is where AI agent hype meets practical value. While some agents fail under unrealistic expectations, others demonstrate real potential in supporting and enhancing business processes.
Key Business Applications
AI agents are particularly valuable in workflow automation, especially for small teams and solo entrepreneurs. They help streamline:
- Scheduling and calendar management
- Inbox organization and email triage
- Customer interaction (via chatbots or virtual assistants)
🔍 Example: According to DeepSense.ai, industries with repetitive yet essential workflows—like onboarding, content moderation, and customer support—see notable efficiency gains from AI agent integration.
By offloading these repetitive tasks, organizations can free up human resources to focus on:
- High-level strategic thinking
- Creative problem-solving
- Personalized customer care
Practical Limitations
Despite the promise, AI agents face several challenges that can hinder successful deployment:
- Unpredictability in real-world environments
- Lack of contextual or cultural understanding
- Difficulty with nuanced communication and emotional tone
- Dependence on extensive training data and tuning
- Limited trust for handling sensitive or high-risk tasks
Austin Starks articulates a critical stance in his Medium article, arguing that AI agents frequently:
- ❌ Fail when nuanced human judgment is required
- ❌ Require time-intensive oversight and fine-tuning
- ❌ Cannot be fully relied on for sensitive or high-stakes interactions
A Balanced Perspective
While AI agents bring growing practical value, businesses must temper optimism with cautious planning. Success requires:
- Informed, strategic adoption
- Clear alignment with actual operational needs
- Avoidance of overreliance on full autonomy
- Continuous performance evaluation
AI agents aren't silver bullets—but when used wisely, they can be powerful tools in the modern business toolbox.
Determining If AI Agents Make Strategic Sense for Your Business
Before embracing AI agents, the key is to establish clear, pragmatic guidelines that allow you to objectively evaluate their fit and feasibility against your existing workflows. Below are two essential parameters to consider:
Scale and Complexity of Tasks
AI agents often shine in scenarios involving tasks characterized by varied complexity or shifting contexts. If your business operations deal with dynamic environments—such as responsive customer service conversations, creative content planning, or adaptive scheduling scenarios—then AI-driven autonomy can introduce meaningful productivity gains. Conversely, if tasks are repetitive, stable, and predictable—such as straightforward bookkeeping or email forwarding—a simpler, linear automation solution proves more cost-effective and dependable.
Flexibility versus Stability
Assess your business's operational priorities: Is flexibility and adaptability paramount, or is reliability and consistency more crucial? Consider an example scenario where flexibility is vital: Suppose your growing consultancy needs to dynamically manage incoming requests, route conversations intelligently, and proactively adjust project timelines. In this case, AI agent integration can be a powerful enabler of growth and responsiveness that static automation could not easily replicate.
On the other hand, if your e-commerce fulfillment process demands consistent, predictable, and replicable steps for order fulfillment and updating inventory databases, traditional linear automation tools or simpler integrations will likely outperform autonomous agents in both dependability and lower maintenance demands.
By carefully evaluating these factors, your business can strategically determine when the adaptive capabilities and complexities of AI agents clearly justify investment, versus when traditional automation tools provide a more reliable and efficient solution.
Practical AI Agent Tools to Explore
As the AI agent ecosystem expands, multiple tools have emerged, crafted to suit various business needs from light task automation to complex autonomous interactions. Below is a clear overview of reputable solutions, each noted for particular strengths to help make informed decisions:
-
1. Auto-GPT (Open Source GPT-based Agent)
Widely recognized for its versatility, Auto-GPT excels at breaking complex tasks into smaller AI-driven steps, autonomously planning and managing workflows. Especially useful for entrepreneurs or small tech teams exploring scalable task automation or concept prototyping through autonomous exploration. -
2. AgentGPT (User-friendly Autonomous GPT Framework)
Offering simplicity and accessibility, AgentGPT allows nontechnical users to quickly spin up easy-to-manage autonomous agents. Ideal for workflow test cases, rapid prototyping, and small business validation scenarios requiring minimal technical overhead. -
3. BabyAGI (Task Management & Autonomous Goal-Setting)
Emphasizing robust iterative task management, BabyAGI is best suited to business use-cases requiring frequent check-ins, proactive reminders, and task reprioritization. Effectively applied in project management settings and productivity monitoring. -
4. LangChain (Flexible AI Agent Toolkit)
Operating as both a framework and interface layer, LangChain helps businesses integrate diverse conversational and analytical AI agents smoothly within existing applications or workflows. Ideal for teams comfortable with development and needing a flexible, customizable toolkit. -
5. SuperAGI (Advanced Autonomous Agents Platform)
Positioned as an enterprise-grade platform, SuperAGI provides a centralized control panel for deploying, monitoring, and fine-tuning AI agents at scale. Recommended for companies looking towards larger deployments and willing to invest significant setup and configuration resources.
By assessing the complexity, scale, and resources devoted to integrating AI agents, business users can confidently navigate diverse choices and strategically employ these advanced tools towards genuine productivity and value gains.
Final Thoughts on the Future of AI Agents
Despite lingering reliability challenges and instances of exaggerated marketing, it remains critical not to dismiss AI agents outright. Their current perceived shortcomings are not indicative of their true long-term potential. As technology evolves, AI agents will undoubtedly become increasingly capable, supportive, and responsive—offering genuine productivity boosts and automating complex workflows in nuanced, contextually aware ways impossible using traditional automation.
Yet, embracing AI agents doesn't mean joining a blind hype parade. Instead, it's about thoughtfully planned experimentation combined with strategic insight:
- Start small, monitor results closely, and proactively assess real-world benefits against clearly defined expectations.
- Embrace intelligent risk-taking by piloting AI solutions within controlled, manageable frameworks.
- Allow data and tangible experience—not hype alone—to guide your progression, identifying practical, measurable improvements in workflow efficiency.
Ultimately, AI agents represent powerful tools potentially capable of reshaping productivity and workflow management, but only when implemented strategically. Make thoughtful, data-driven decisions, and stay alert to developments—ensuring your investment and adoption remain aligned clearly with your organizational goals, resources, and practical outcomes, not fleeting market trends.
Book a Free Consultation to Optimize Your AI Workflow
Navigating the dynamic landscape of AI agents and automation can seem overwhelming. If you're exploring the potential of integrating AI into your business workflow, clarity and unbiased expertise can make the difference between fruitful innovation and costly experimentation.
We're here to offer professional guidance tailored precisely to your situation—whether you're a solo entrepreneur, managing a small team, or scaling up more complex operations. Our consultation provides clear, practical advice that cuts through the hype, identifies real opportunities, and realistically assesses challenges.
During your free session, we'll discuss:
- How AI agents might directly benefit your existing workflows.
- Realistic assessments of complexity versus strategic advantages.
- Best practices for combining reliable linear automation with flexible AI assistance.
- Strategies to maintain control, ensure reliability, and measure meaningful gains in efficiency.
Reach out today, and stop guessing about AI. Take informed action powered by insights and clear strategy.
Ready to explore intelligent, reliable ways to streamline and automate with AI agents? Book your free consultation session today.